Blog Post
How to Choose the Optimal CRQ Framework
November 22, 2022
TL;DR
- Cyber risk quantification (CRQ) helps organizations prioritize security efforts by translating risks into potential financial losses.
- Kovrr’s CRQ framework leverages real threat intelligence, insurance claims, and internal data to accurately estimate those losses.
- The platform consolidates diverse inputs into a unified model and runs simulations to project likely cyber events and their costs.
- This approach reduces guesswork, improves decision-making, and provides clear justification for cybersecurity investments.
- Kovrr’s unique methodology is fast, automated, and built for repeated use, making it practical for real-time cyber risk management.
To get a handle on increased cybersecurity threats, businesses need to know what’s at stake. If you don’t know what you’re defending and what the implications of a cyber event could be, then it’s hard to make cybersecurity decisions.
For example, you could be spending time and money on cybersecurity awareness training while your biggest vulnerabilities stem from third-party exposure. Conversely, you might be focusing on adding cybersecurity software, but if employees are unknowingly letting attackers waltz in, then maybe cybersecurity awareness training would do more to reduce your risk.
In other words, cyber risk management strategies are context-dependent. What works best at one organization might not be the best approach for another. That’s why companies need to conduct cyber risk quantification (CRQ) so they can know what their full exposure to cyber events looks like.
Yet there are several different types of CRQ frameworks to choose from. While it’s generally better to conduct some type of CRQ rather than none at all, due to the ongoing changes in the cyber risk landscape, it makes the most sense to utilize an option that allows your cybersecurity team to run a cyber risk quantification often and on-demand., rather than taking a one-time snapshot.
You’ll also likely want one that’s rooted in a methodology that gives you confidence in following recommendations that stem from the analysis on how to improve your cyber posture and decrease financial exposure.
What Is a CRQ Framework and Why Use One?
A CRQ framework is a process for quantifying cyber risk. Typically, Kovrr uses the definition of cyber risk quantification that means assigning a dollar amount to different cyber risks. That’s important because if you know that some types of cyber events could result in a $100,000 loss, while other types of cyber events expose your organization to $1 million in losses, you’re probably going to prioritize the larger amount.
But CRQ can also involve other types of quantification. For example, you might work with a consultant to assign scores to different areas of risk for your business. Or you might quantify issues like the number of endpoints into your network.
However, these types of frameworks can be more limiting. If you don’t know what the financial implications of a cyber attack could be, then you don’t necessarily know how to prioritize cyber risk management.
For example, your data security practices might score low, but maybe your organization’s data has limited financial value on a relative basis. Instead, you could be facing larger losses if your operations were to come to a halt due to ransomware, for example.
If a CRQ framework can help you figure out what your loss exposure looks like, then you can take steps to remedy these areas, like investing in relevant cybersecurity tools or changing employee practices. You can’t always tackle all risks at once, so it often makes sense to focus on those that could lead to the highest costs.
Plus, understanding the financial implications of a cyber event can help you assess the ROI of cyber investments. If you’re weighing whether to add cyber insurance, for example, you can use CRQ to get a better sense of what your losses could look like.
As a TechCrunch headline proclaims, “2023 will be the year of cyber-risk quantification.” Still, some CRQ frameworks could be better suited to your needs than others, so it’s important to choose the right one.
What Differentiates Kovrr’s CRQ Framework?
Kovrr’s Data Sources
Proprietary data collected by Kovrr provides a facts-based view of threats, variabilities, and the actual costs of cyber events. The data enables Kovrr to create accurate inherent risk based on company industry size and location.
Modeling impacts from cyber events requires an extensive understanding of the cyber threat landscape. A core aspect of Kovrr’s data pipeline combines unique data sources to better inform the data points taken into account when building out the frequency and severity of cyber events.
Kovrr’s data collection streams dozens of sources into Kovrr’s threat intelligence and cyber events databases. The data sources can be grouped into several categories. More info on our data sources can be found in this article on threat intelligence data.
Another important data source is claims data. Over time, Kovrr has formed data-sharing partnerships with some of the leading players in the cyber insurance market (primary insurance carriers, reinsurance carriers, and reinsurance brokers). This has allowed Kovrr to obtain two critical and data-rich inputs to inform the model severity functions- losses based on individual claims data and aggregated yearly claims losses based on industry location and revenue bands of the companies.
Individual claims usually include details about the incidents in addition to the costs: what happened and how it affected the business, along with more information about the direct costs associated with the incidents.
These costs include the cost of incident response and forensics, recovery, loss of revenue, penalties, and fines, legal defense, PR repair, notification costs, and monitoring services.
The framework is also based on an insurance-validated modeling methodology. We incorporate real data from insurance carriers and enterprises to continually improve the CRQ accuracy. As more data becomes available, you can get a better understanding of what different types of cyber events could translate to in financial terms.
Kovrr’s Methodology
One of the biggest challenges of cybersecurity is the ability to consolidate and integrate different types of data into one coherent analysis and decision plan. Most companies implement different types of solutions, such as endpoint detection and response (EDR), SIEM solutions, IDS/IPS, Breach Attack Simulation (BAS), Attack Surface Management tools (ASM), and many more. In addition to all these tools and solutions, other data sources external to security tools are needed, for example, threat intelligence, industry-level threats, and real-time events.
The result of all of these tools is usually a vast amount of data, but not necessarily a coherent and consistent view of risk. This makes it difficult to understand what should be done to mitigate or address the risk in the most impactful way because different recommendations, actions, and risk views arise from each data source. Trying to reach a practical plan from all the data sources, can be time-consuming and tedious since it requires analyzing a vast amount of data and translating it all to one unified language.
The same challenge also applies when performing cyber risk quantification. The accuracy of the quantification lies in the ability to take into account different sources that help in understanding different aspects of the risk. This is why Kovrr uses multiple data source types such as data integrations, threat intelligence, the cyber sphere, and the External Scan. Once all data types are collected, they are consolidated into one normalized data layer, via Kovrr’s consolidator, that becomes the baseline for the analysis.
The consolidation represents Kovrr's general approach to cyber quantification, which requires a 360° view of risk. A valid quantification needs to take into account the cyber data about the company and cross-reference it with the industry data along with the cyber context in which the company operates.
Consolidating data has 3 main challenges:
- Attribution. The consolidator detects the same entities that are covered by different data sources and attributes them as the same entity. This allows us, for example, to be able to connect between an asset that is identified by the user using the cyber sphere, to vulnerabilities that are associated with the same asset from a vulnerability management tool. This creates a commonly based company map based on all data sources and defined in the same language.
- Multi-type. The consolidator needs to handle data types that are fundamentally different, ranging from the user-defined cyber sphere to vulnerability management platform outputs to threat intelligence data and more. Each data type is treated as a plug-in for the consolidator. Once mapped, each data type is standardized to the same internal modeling framework.
- Inference. Some of the data that is consolidated does not appear in the data sources explicitly and directly, but rather needs to be inferred, i.e; if an inference is security controls. Whilst data about security controls can sometimes be explicit (e.g. in the cyber sphere), it is mostly inferred in the case of vulnerability management tools. The presence of a vulnerability, or other security problem, indicates that a security measure is lacking, and therefore there is a missing security control.
Once we have all the data needed for the simulation consolidated, and after the consolidation step, we build a catalog of events to test likely attacks against the company. This event catalog is later used in a Monte-Carlo Simulation, in which we simulate cyber events, and for each event, we calculate the cost to the business.
The simulation is based on a Monte Carlo simulation, in which we simulate the following year. The events are then used in our bespoke event catalog. The events are grouped into annual scenario groups, allowing the platform to test the impact of each event and the likely combinations of events that can hit a company within the same year. Each scenario year will have a number of cyber events occurring, some will have no events, and some will have multiple events.
The foundation of the quantification is based on calculating the losses associated with each of the cost components. The financial impact of each simulated event is derived by summing the losses from the relevant cost components per event. Once the loss from each event is known, it can then be applied to the entire simulation.
A mix of validation tests and risk controls are applied throughout the model development process to limit model risk, ensure the correct operation of the model, and maintain the quality of the model.
The three main areas of validation and testing are: Validation, Parameter Testing, and Model Calculation Testing.
If you’re deciding between different frameworks, we invite you to consider the time and personnel resources it would take to conduct more manual approaches vs. the more automated, detailed, non- biased financial modeling that our framework provides.
See for yourself with a free demo on how Kovrr can help you improve cyber risk management.
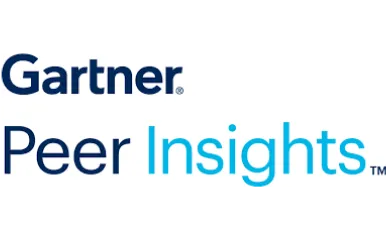

